Explainable AI: Making Artificial Intelligence Transparent and Trustworthy
Artificial Intelligence (AI) has become a transformative force across industries, but its complex and often opaque decision-making processes raise questions about trust and accountability. Explainable AI (XAI) seeks to address these concerns by making AI systems more transparent, enabling users to understand and trust their outputs. In this article, we explore the principles of XAI, its importance, and its applications in critical sectors.
What is Explainable AI?
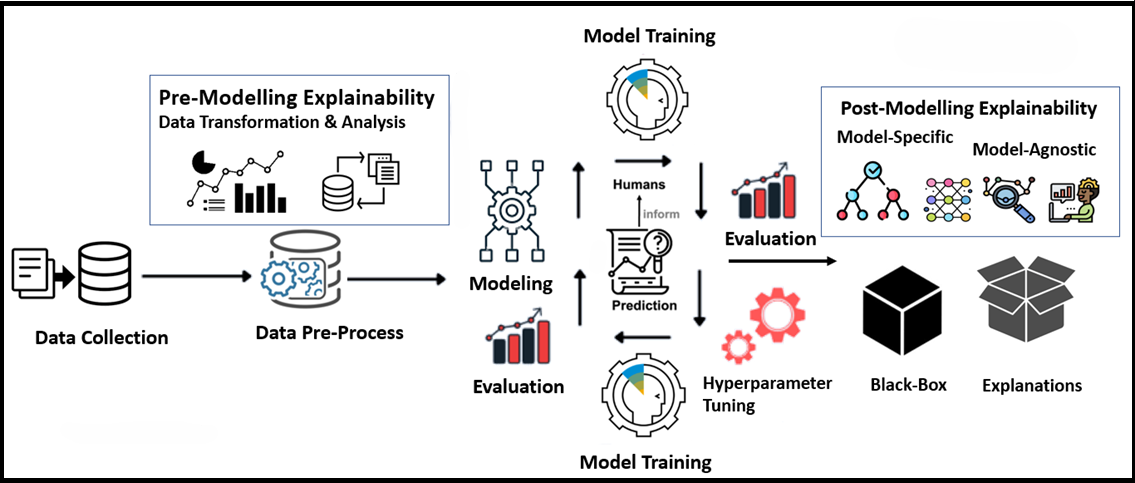
Explainable AI refers to methods and techniques that make the inner workings of AI systems understandable to humans. Unlike traditional AI, where decision-making processes are often considered "black boxes," XAI focuses on transparency by providing explanations for decisions made by AI models. This enhances trust and ensures accountability, especially in high-stakes domains.
Why is Explainability Important?
- Trust and Accountability: Users and stakeholders need to trust AI systems, especially in sensitive areas like healthcare and finance.
- Compliance with Regulations: Explainability is critical for meeting legal and ethical standards, such as the EU’s General Data Protection Regulation (GDPR).
- Debugging and Improvement: Understanding AI behavior helps developers identify errors and refine models.
Applications of Explainable AI
1. Healthcare
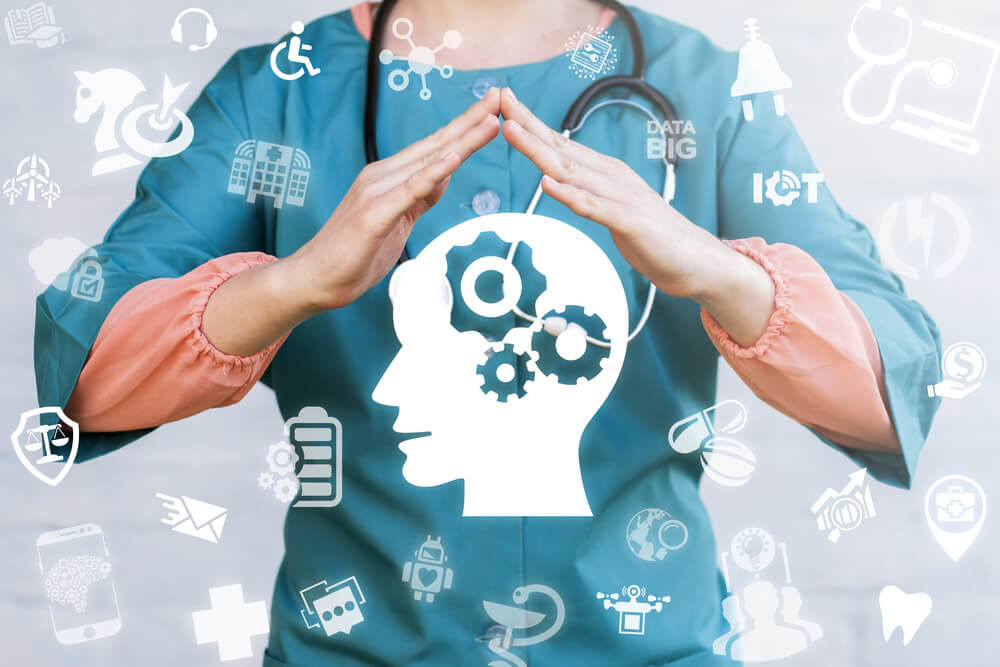
In healthcare, XAI provides doctors and patients with understandable explanations of AI-driven diagnoses and treatment recommendations. For example:
- Medical Imaging: AI tools like IBM Watson Health explain anomalies in scans to support clinical decisions.
- Drug Discovery: XAI helps researchers understand why specific molecules are selected for trials.
2. Finance
XAI improves transparency in automated credit scoring and fraud detection systems. By explaining decisions like loan approvals or flagged transactions, financial institutions can ensure fairness and build customer trust.
3. Law Enforcement
AI systems used in predictive policing or risk assessment benefit from explainability to address ethical concerns. By revealing the rationale behind decisions, XAI reduces bias and promotes accountability.
Challenges in Explainable AI
Despite its potential, XAI faces challenges such as:
- Balancing Complexity and Simplicity: Making explanations understandable without oversimplifying technical details.
- Scalability: Ensuring XAI techniques are scalable across large datasets and complex models.
- Domain-Specific Needs: Tailoring explanations to suit specific industries and user requirements.
Conclusion
Explainable AI is a crucial step toward building trustworthy, ethical, and effective AI systems. By bridging the gap between complex algorithms and user understanding, XAI not only enhances trust but also ensures AI's responsible integration into society. As AI adoption grows, explainability will remain central to its future development and success.